Multi-Scale Deep Residual Learning-Based Single Image Haze Removal via Image Decomposition
Haze in images captured under adverse weather conditions can significantly degrade visual quality and negatively impact performance in outdoor visual surveillance and other applications. This paper proposes a novel framework called MSRL-DehazeNet (Multi-Scale Residual Learning Dehazing Network) for single image haze removal. Our approach reformulates the problem as restoration of the image base component through image decomposition, rather than using end-to-end mapping between hazy and haze-free images.
Research Focus
- A image decomposition strategy separating hazy images into base and detail components
- Three specialized components:
- Multi-scale deep residual learning for haze-free base component restoration
- Simplified U-Net structure to avoid color distortion in recovered images components
- CNN-based detail enhancement
Proposed Architecture
MSRL-DehazeNet
Architecture
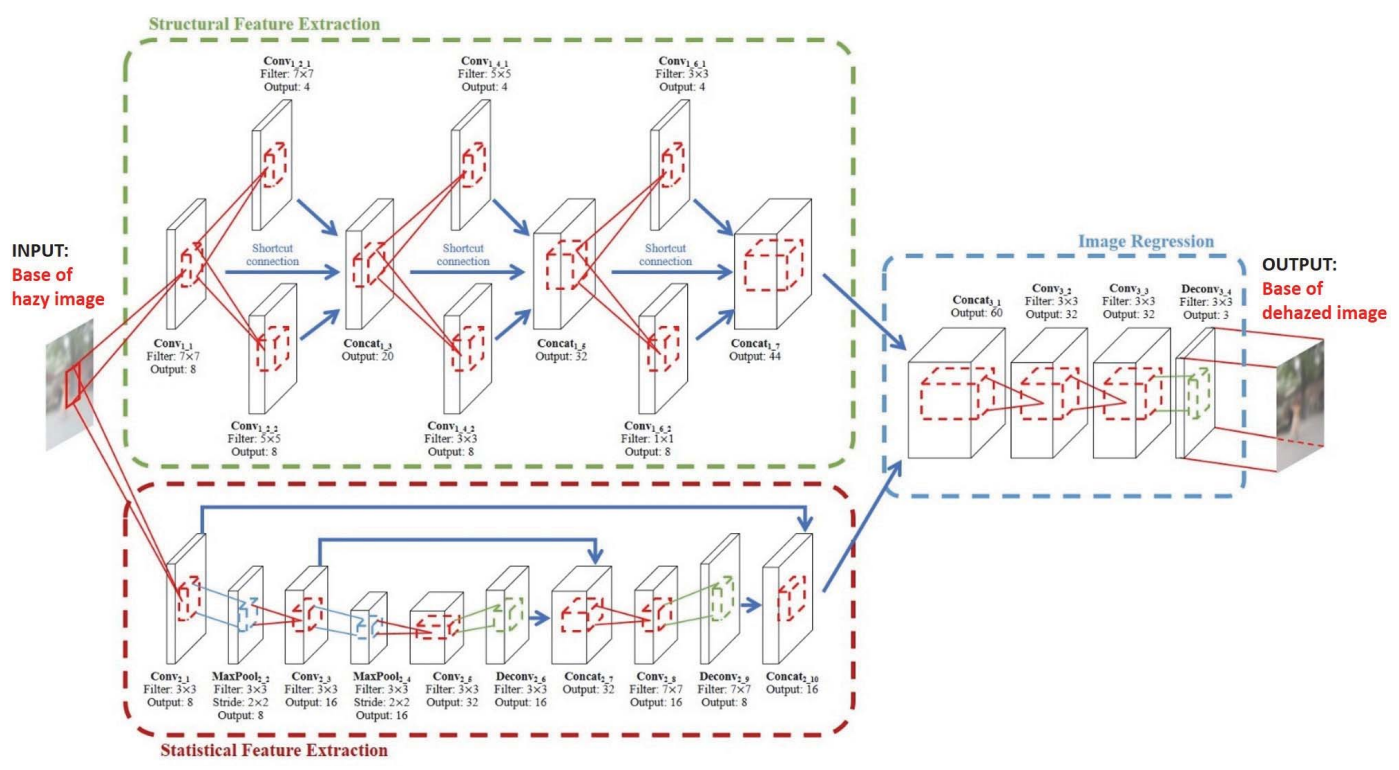
Network Visualization
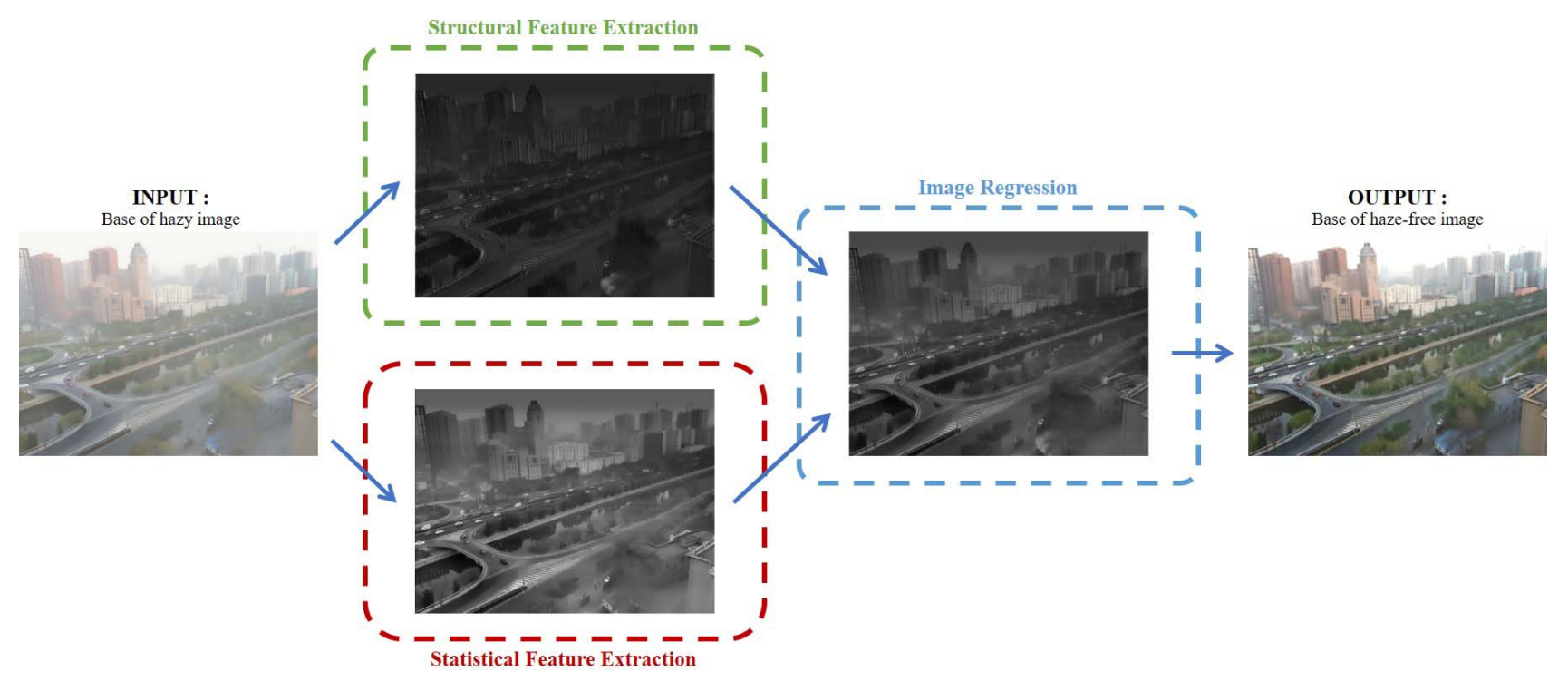
Factor Prediction CNN
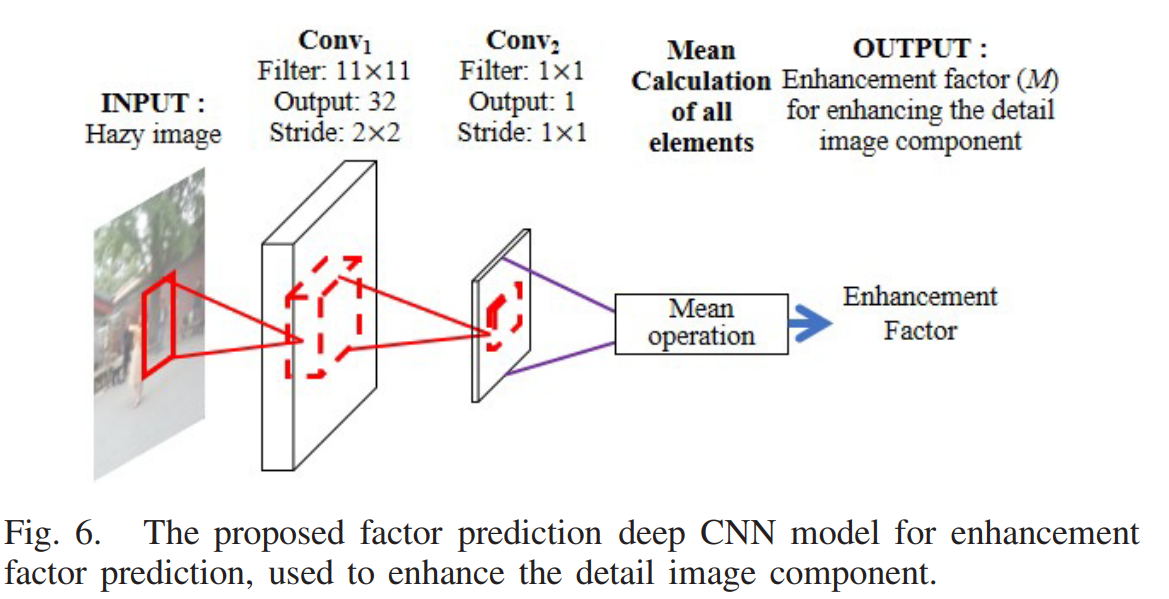
Performance Evaluation
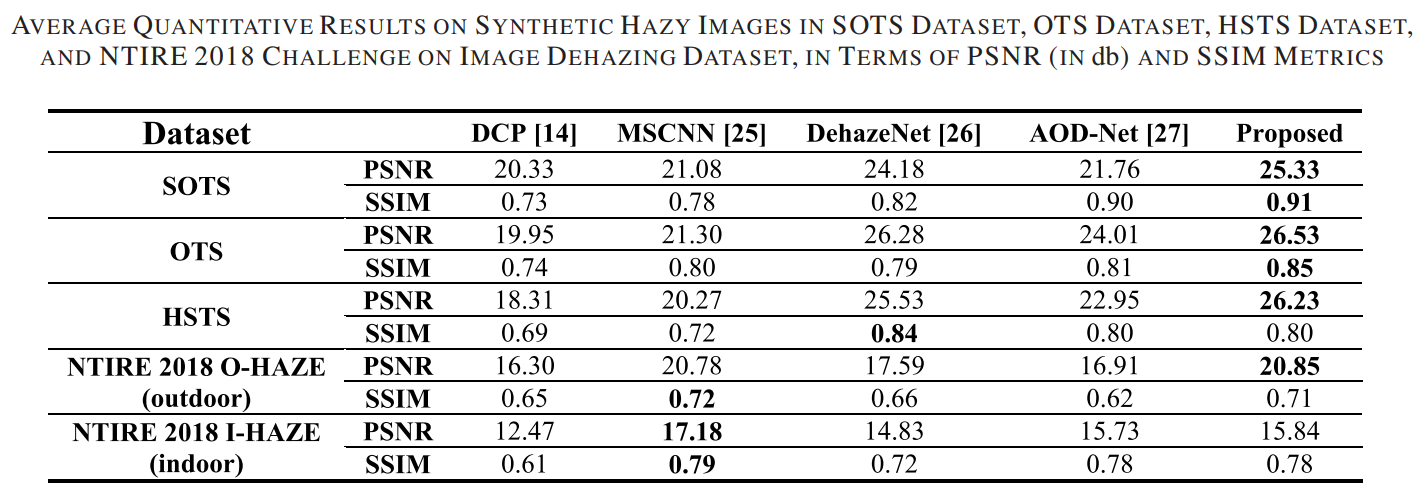
Experimental Results
SOTS dataset
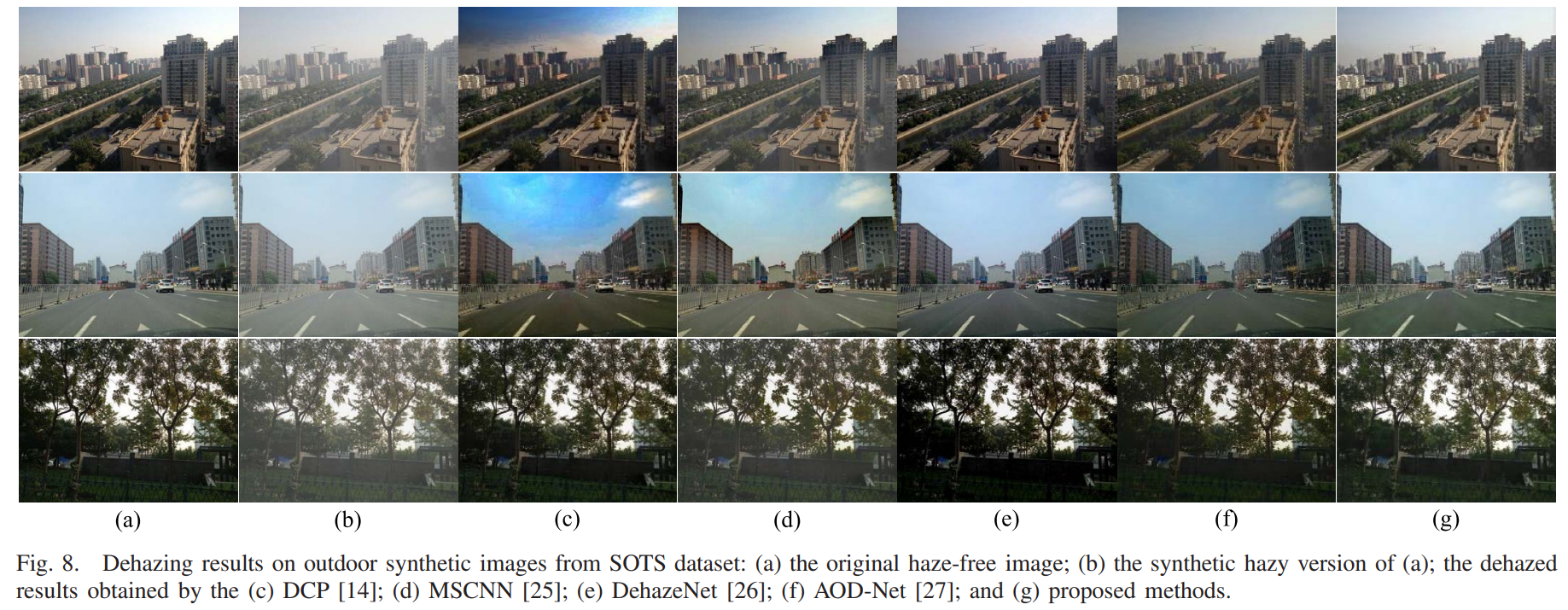
OTS dataset
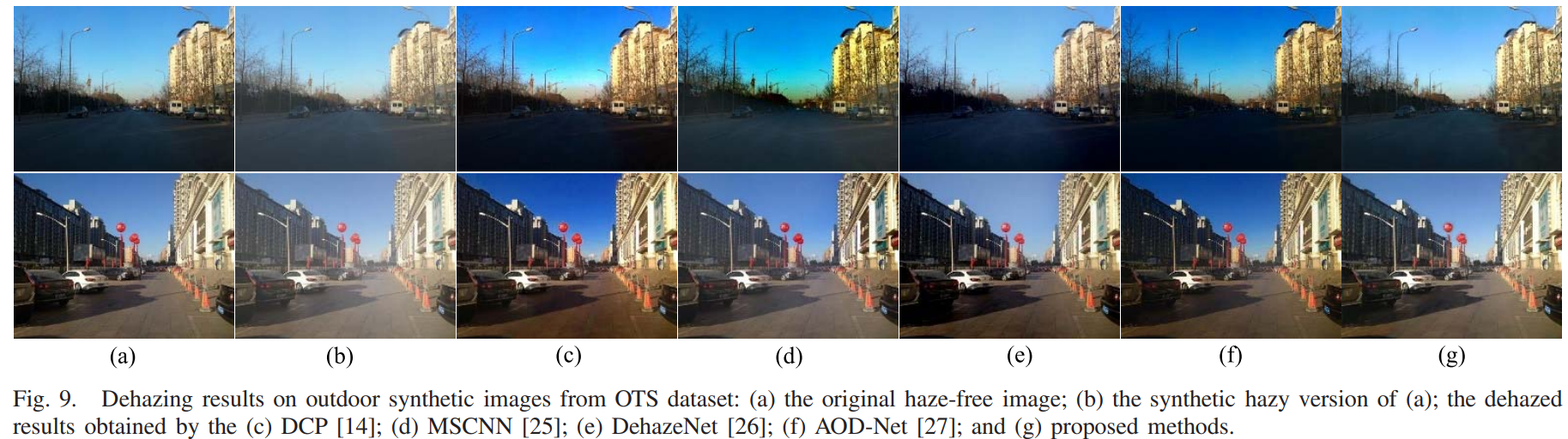
HSTS dataset
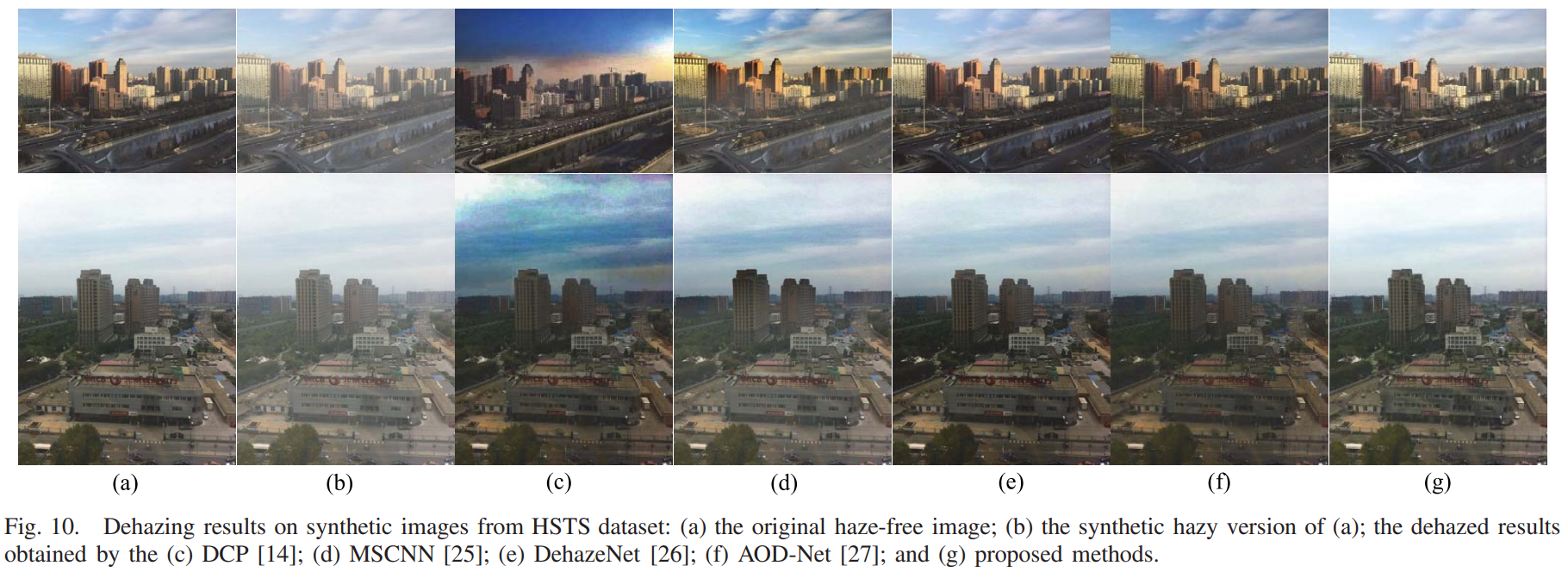
Run-time Result
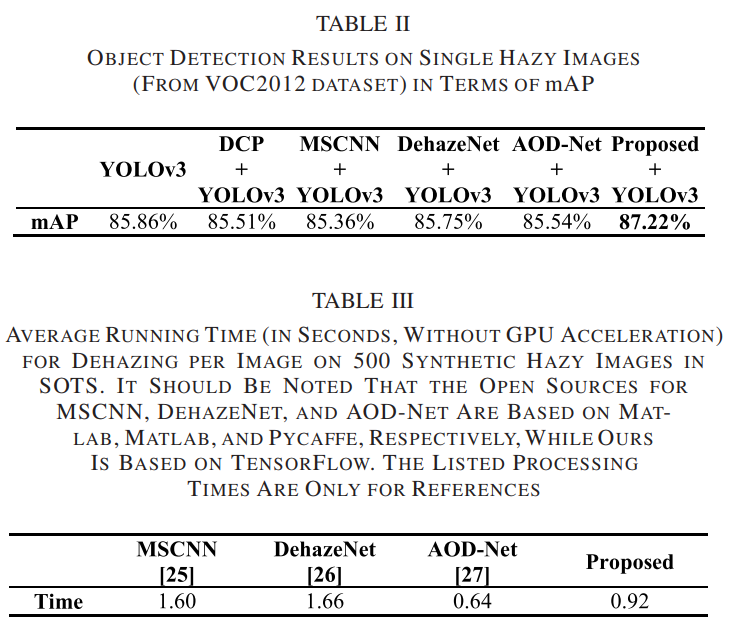